Concepts#
Engine#
The essence of the framework is the class Engine
, an abstraction that loops a given number of times over
provided data, executes a processing function and returns a result:
while epoch < max_epochs:
# run once on data
for batch in data:
output = process_function(batch)
Thus, a model trainer is simply an engine that loops multiple times over the training dataset and updates model parameters. Similarly, model evaluation can be done with an engine that runs a single time over the validation dataset and computes metrics. For example, model trainer for a supervised task:
def update_model(trainer, batch):
model.train()
optimizer.zero_grad()
x, y = prepare_batch(batch)
y_pred = model(x)
loss = loss_fn(y_pred, y)
loss.backward()
optimizer.step()
return loss.item()
trainer = Engine(update_model)
trainer.run(data, max_epochs=100)
Events and Handlers#
To improve the Engine
’s flexibility, an event system is introduced that facilitates interaction on each step of
the run:
engine is started/completed
epoch is started/completed
batch iteration is started/completed
Complete list of events can be found at Events
.
Thus, user can execute a custom code as an event handler. Let us consider in more detail what happens when
run()
is called:
fire_event(Events.STARTED)
while epoch < max_epochs:
fire_event(Events.EPOCH_STARTED)
# run once on data
for batch in data:
fire_event(Events.ITERATION_STARTED)
output = process_function(batch)
fire_event(Events.ITERATION_COMPLETED)
fire_event(Events.EPOCH_COMPLETED)
fire_event(Events.COMPLETED)
At first engine is started event is fired and all this event handlers are executed (we will see in the next paragraph how to add event handlers). Next, while loop is started and epoch is started event occurs, etc. Every time an event is “fired”, attached handlers are executed.
Attaching an event handler is simple using method add_event_handler()
or
on()
decorator:
trainer = Engine(update_model)
trainer.add_event_handler(Events.STARTED, lambda engine: print("Start training"))
# or
@trainer.on(Events.STARTED)
def on_training_started(engine):
print("Another message of start training")
# attach handler with args, kwargs
mydata = [1, 2, 3, 4]
def on_training_ended(engine, data):
print("Training is ended. mydata={}".format(data))
trainer.add_event_handler(Events.COMPLETED, on_training_ended, mydata)
Note
User can also register custom events with register_events()
, attach handlers and fire custom events
calling fire_event()
in any handler or process_function.
See the source code of create_supervised_tbptt_trainer
for an example of usage of
custom events.
Timeline and events#
Below the events and some typical handlers are displayed on a timeline for a training loop with evaluation after every epoch:
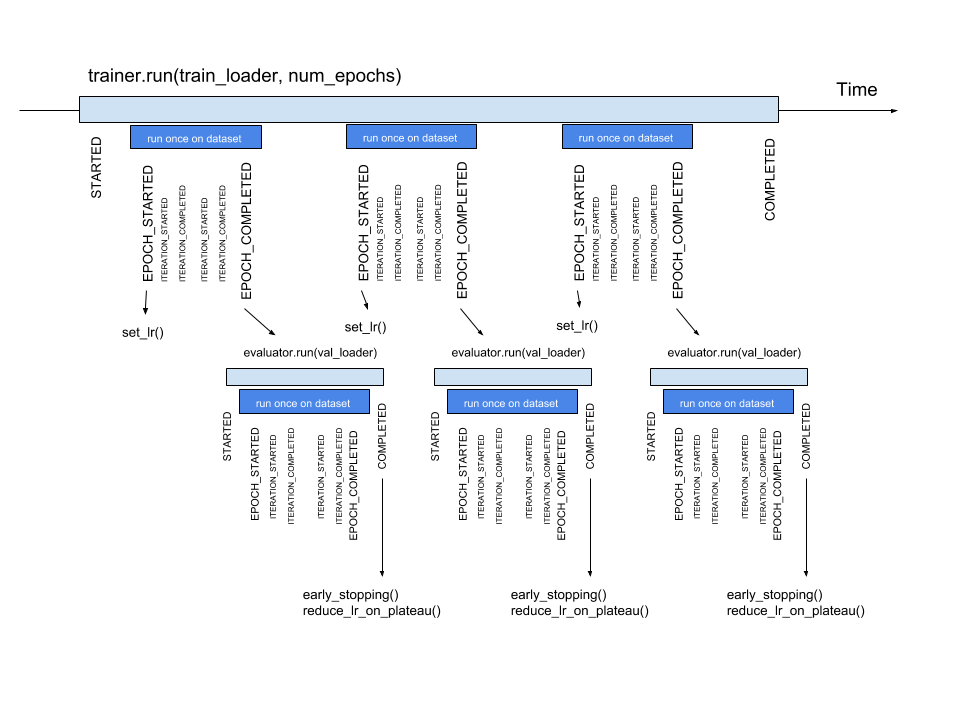
State#
A state is introduced in Engine
to store the output of the process_function, current epoch,
iteration and other helpful information. Each Engine
contains a State
,
which includes the following:
engine.state.epoch: Number of epochs the engine has completed. Initializated as 0.
engine.state.max_epochs: Number of epochs to run for. Initializated as 1.
engine.state.iteration: Number of iterations the engine has completed. Initialized as 0.
engine.state.output: The output of the process_function defined for the
Engine
. See below.
In the code below, engine.state.output will store the batch loss. This output is used to print the loss at every iteration.
def update(engine, batch):
x, y = batch
y_pred = model(inputs)
loss = loss_fn(y_pred, y)
optimizer.zero_grad()
loss.backward()
optimizer.step()
return loss.item()
def on_iteration_completed(engine):
iteration = engine.state.iteration
epoch = engine.state.epoch
loss = engine.state.output
print("Epoch: {}, Iteration: {}, Loss: {}".format(epoch, iteration, loss))
trainer.add_event_handler(Events.ITERATION_COMPLETED, on_iteration_completed)
Since there is no restrictions on the output of process_function, Ignite provides output_transform argument for its
metrics
and handlers
. Argument output_transform is a function used to transform engine.state.output for intended use. Below we’ll see different types of engine.state.output and how to transform them.
In the code below, engine.state.output will be a list of loss, y_pred, y for the processed batch. If we want to attach Accuracy
to the engine, output_transform will be needed to get y_pred and y from
engine.state.output. Let’s see how that is done:
def update(engine, batch):
x, y = batch
y_pred = model(inputs)
loss = loss_fn(y_pred, y)
optimizer.zero_grad()
loss.backward()
optimizer.step()
return loss.item(), y_pred, y
trainer = Engine(update)
@trainer.on(Events.EPOCH_COMPLETED)
def print_loss(engine):
epoch = engine.state.epoch
loss = engine.state.output[0]
print ('Epoch {epoch}: train_loss = {loss}'.format(epoch=epoch, loss=loss))
accuracy = Accuracy(output_transform=lambda x: [x[1], x[2]])
accuracy.attach(trainer, 'acc')
trainer.run(data, max_epochs=10)
Similar to above, but this time the output of the process_function is a dictionary of loss, y_pred, y for the processed batch, this is how the user can use output_transform to get y_pred and y from engine.state.output. See below:
def update(engine, batch):
x, y = batch
y_pred = model(inputs)
loss = loss_fn(y_pred, y)
optimizer.zero_grad()
loss.backward()
optimizer.step()
return {'loss': loss.item(),
'y_pred': y_pred,
'y': y}
trainer = Engine(update)
@trainer.on(Events.EPOCH_COMPLETED)
def print_loss(engine):
epoch = engine.state.epoch
loss = engine.state.output['loss']
print ('Epoch {epoch}: train_loss = {loss}'.format(epoch=epoch, loss=loss))
accuracy = Accuracy(output_transform=lambda x: [x['y_pred'], x['y']])
accuracy.attach(trainer, 'acc')
trainer.run(data, max_epochs=10)
Note
A good practice is to use State
also as a storage of user data created in update or handler functions.
For example, we would like to save new_attribute in the state:
def user_handler_function(engine):
engine.state.new_attribute = 12345